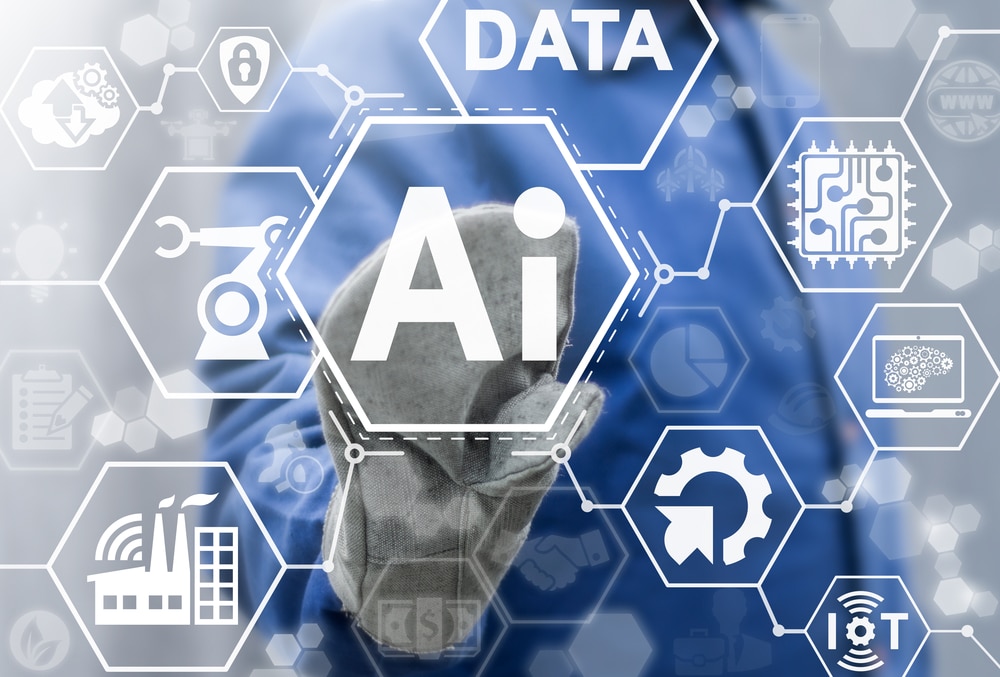
Accelerating Drug Discovery and Development with Artificial Intelligence
With the rate of data generation increasing exponentially in today’s technology-driven R&D world, there are both enormous opportunities and real challenges for science-driven organizations in transforming this data into pragmatically useful insights. For example, in the biopharmaceutical industry, Next Generation Sequencing screening technologies generate terabytes of data in a single run and automated bioreactors create multiple streams of process control and analytical data requiring highly integrated analysis. Additionally, growing datasets from patients, caregivers, and other sources, are creating massive repositories of data that researchers can exploit to identify and optimize potential new drug candidates.
This explosion in both the types and volume of data is such that the ability of the human brain to process and evaluate the data is stretched to its capacity. In order to efficiently extract useful information from the data, the industry has been adopting artificial intelligence (AI) and big data approaches, particularly machine learning and deep learning. Numerous areas of biopharmaceutical R&D activity throughout discovery, clinical development, risk assessment, safety monitoring, regulatory, and manufacturing are turning to techniques developed for “big data” problems in the financial and consumer industries.
According to the consulting firm CB Insights, $3.6 billion was invested across 481 healthcare AI deals from the first quarter of 2013 through the first quarter of 2018. Just a few of the many recent partnerships between major pharmaceutical companies and AI startups include:
- In April 2019, AstraZeneca started a partnership with BenevolentAI to help identify and develop new drugs for chronic kidney disease and idiopathic pulmonary fibrosis.
- In March 2019, Bristol-Myers Squibb (BMS) announced a multi-year strategic agreement with Concerto HealthAI that will integrate multiple data sources and apply AI and machine learning to accelerate oncology clinical trials, enable robust protocol design and generate insights for precision treatment and improved patient outcomes.
- In June 2019, Atomwise announced a multi-year collaboration with Lilly to apply Atomwise’s patented artificial intelligence (AI) technology in support of Lilly’s preclinical drug discovery efforts.
These are some recent examples of the optimism that these technologies will change the way the biopharmaceutical industry discovers, develops, and manufactures medicines. In this blog, we will focus specifically on the challenges inherent in traditional drug discovery and development and discuss some of the ways in which biopharmaceutical companies are utilizing AI to improve efficiencies and speed the process of bringing new drugs to market.
Drug Discovery and Development Challenges
Drug discovery and development are challenging tasks. According to a 2014 study sponsored by the US Department Health and Human Services, it costs somewhere between $161 million and $2 billion to bring a new drug to market. This study also concluded that the average length of time from clinical development to marketing is on the rise over the last decade and currently stands at about 7.5 years. Including the discovery phase, this puts the time from initial R&D to market for a new drug well over a decade. As these longer timelines mean increased costs and decreased revenue, there is intense pressure to speed time to market for new drugs.
A study on drug development success rates from 2006-2015 revealed that only 10% of drug candidates entering Phase I clinical trials make it to FDA approval stage. These poor success rates are a key component of the costs of drug discovery and development, and additionally these costs get passed down to patients, which can make drugs inaccessible to those who are uninsured or underinsured.
It’s also important to note that an FDA approval is by no means a guarantee that a company will see a return on their investment. Post-market safety issues, competitive drug product releases, the increased speed of generic development, and market cost pressures all impact the economics of drug discovery and development.
How AI Can Accelerate Drug Discovery and Development
There are several areas where AI is being applied to improve the drug discovery and development process. A few of these include:
Drug Candidate Identification. One of the most time-intensive steps in drug discovery is identifying which of the thousands of available chemical compounds can potentially offer help in treating an illness. The painstaking process of screening all these molecules for drug candidates takes enormous amounts of time for human researchers. Artificial intelligence has the potential to more rapidly and thoroughly analyze the reams of data produced in drug discovery to predict which drug candidates are likely to be effective treatments, saving both time and money for biopharmaceutical companies, as well as extracting more value out of the data generated by experimentation.
GlaxoSmithKline (GSK) is one of the companies taking the lead in this area. They announced a strategic drug discovery partnership utilizing Exscientia’s AI-enabled platform in 2017 to discover novel and selective small molecules for up to 10 disease-related targets. GSK is hopeful that this partnership will result in significantly shorter development times and reduced costs, as drug discovery screenings will mostly be replaced by supercomputer simulations and predictive algorithms. The first milestone in this collaboration was achieved in 2019, when Exscientia delivered “a highly potent in vivo active lead molecule targeting a novel pathway for the treatment of chronic obstructive pulmonary disease (COPD).”
Forecasting Clinical Success. Another barrier to the successful development of new medicines is the high attrition rate of candidate drugs mentioned above. Improving the success percentage by just a few points by having less of these failed candidates would make it to clinical trials in the first place would be worth billions to the industry. By using AI, companies are hoping to better forecast the effects of substances earlier in the development process, effectively preserving clinical trial capacity and resources for testing drugs that have a better chance of success.
The German company Innoplexus AG is making progress in this area. The company says its machine learning software can crawl through as many as 5 billion web pages a day using a natural-language processing algorithm trained on medical research to help assess the probabilities for the outcomes of clinical trials. Internal tests done by Innoplexus on 20,000 completed clinical trials found that their system correctly forecast the outcome of trials around 85% of the time.
Combination Treatments. Finding effective combination therapies is one of the most challenging tasks for researchers. As IBM points out in a recent review, there are 1.8 million new articles published annually in MEDLINE alone, while the average human researcher reads only 250 to 300 medical and scientific journal articles a year. This suggests that human scientists struggle to keep up with all the research going on in their field. In contrast, IBM’s Watson AI supercomputer has “read” 25 million Medline abstracts, more than 1 million full-text medical journal articles, 4 million patents and is regularly updated.
Pfizer announced a collaboration with IBM Watson in late 2016 to assist with finding combinations of agents that will spur the immune system into action and lead to better treatments for cancer. The hope is that Watson help Pfizer make non-obvious connections that its human scientists have missed, as finding combination medicines is even more challenging than looking for single compound therapies.
Creating Personalized Medicines from Genetic Markers. Personalized medicine is based on the ability to identify patient sub-populations thought accurate diagnostic testing for biomarkers. Given the enormous amount of genomics data available, identifying effective biomarkers can be challenging for researchers.
Genentech recently announced a collaboration with Cambridge, MA-based GNS Healthcare whose core focus is tailoring treatments to individuals genes. In this partnership, Genentech will use the GNS REFS™ (Reverse Engineering and Forward Simulation) causal machine learning and simulation platform to look for genetic patient response markers that could lead to targeted therapies. In addition, BenevolentAI – a leader in the use of AI for efficient diagnosis of diseases and drug discovery – recently discovered a number of promising biomarkers in Amyotrophic Lateral Sclerosis (ALS) disease that have produced several candidate drugs currently in development.
Hype versus Reality
It should be noted as a cautionary example that IBM appears to be redirecting its work on IBM Watson for Drug Discovery to clinical trial matching, which has seen more success. This was apparently based on lack of sufficient demand and financial performance for IBM Watson in this space. Similarly, in early 2017, the highly publicized partnership between MS Anderson cancer center and IBM was put on hold.
As with many other examples of new technologies, the potential of AI as applied drug discovery and development is often overstated. With respect to the use of AI, there are significant concerns about the nature of the computer models that are produced with certain techniques: many deep-learning approaches for example produce black-box decisions with little traceability and insufficient supporting information. A cautionary approach to the vendor promises is warranted as we develop a better understanding of the best practices and limitations of AI in drug discovery and development.
Additionally, the application of big data and machine learning have at their core the need for quality data. Preparing the data for use with these techniques – data cleansing – is today the single largest consumer of time and resources in these projects. The ability to recognize, and correct or remove flawed data, is a major consideration in these efforts. This makes a well-designed data architecture an essential pre-requisite. That architecture must cover the full data lifecycle, address master and reference data relationships, provide provenance, and ensure data integrity.
Conclusion
The examples cited in this blog document a few of the ways in which AI can potentially assist biopharmaceutical companies in drug discovery and development. Biopharmaceutical companies are currently exploring uses of this technology in all aspects of operations – from drug R&D to manufacturing, and even within product marketing and patient support. A small sampling of additional ways in which AI could impact the industry includes:
- Finding new uses for previously tested compounds
- Analyzing longitudinal patient data to match patients with the most appropriate long term treatment regimens
- Analyzing at medical images to detect signals the human eye would never see
- Improving clinical trial design, site selection and patient enrollment activities
- Improve the effectiveness and efficiency of pharmacovigilance measures
- Improving patient outcomes with mobile coaching using real-time data collection
- Improving clinician chronic disease management
While AI holds much promise for transforming the Life Sciences, it is by no means a silver bullet. Successfully applying AI is much more than just deploying software. AI techniques will only be as good as the data they are given – clean, robust and large datasets are necessary for AI to generate effective conclusions. Companies that are considering AI solutions must first ensure that their overall systems architecture is capable of providing the data needed.
No one expects AI to replace scientists. Instead, AI will help scientists work smarter by enabling more rapid and complete analysis of much larger data repositories to make decisions. It will always be necessary for human scientists to validate conclusions drawn by AI systems. Ultimately, AI will be a tool that will benefit patients by helping scientists create more and better life-saving medicines.
Case Study: LabWare Centralized Data Review for a Global Biopharmaceutical Company
Overview A global biopharmaceutical company specializing in discovery, development,... LEARN MOREWhite Paper: Managing Data Integrity in FDA-Regulated labs.
New White Paper LEARN MORELET´S GET STARTED
Contact us today and let’s begin working on a solution for your most complex strategy, technology and staffing challenges.
CONTACT US