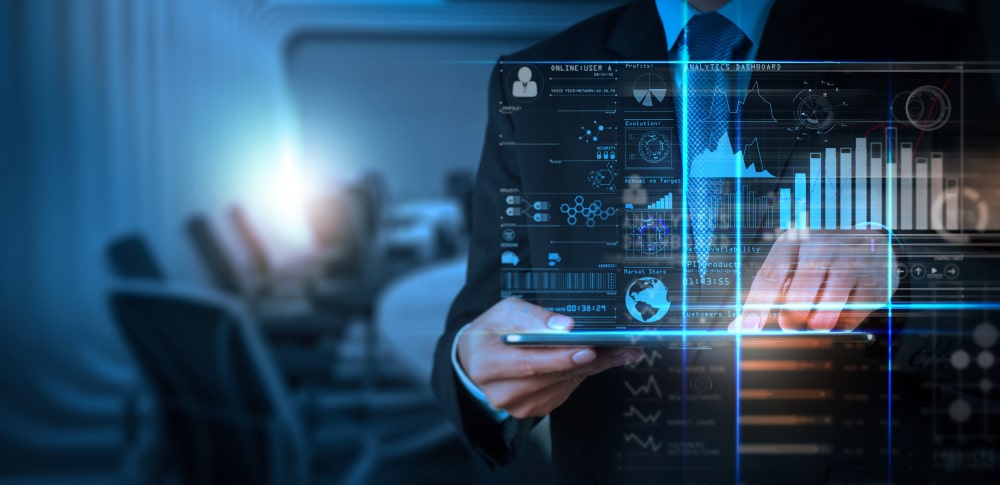
Laboratory Software Spotlight – Riffyn
Digitally Driving Data Analytics for Faster Product Development
Leaders of scientific R&D groups understand that an ecosystem of multi-disciplinary workflows drives innovation, such as high quality drugs and therapeutic agents. To stay competitive, product developers rely on data to make informed decisions. With the growing necessity and complexity of lab informatics technologies, the flow of data and information is also becoming more prone to disconnects. Disparate sources of information give only a sliver of the full data landscape. It can mislead decisions, costing million dollars in lost time, and resources. And worst of all, it can lead a development program down the wrong path.
Riffyn was founded in 2014 to address this issue with the development of the world’s first Process Data System, Riffyn Nexus. This cloud based platform uses R&D and manufacturing processes as the organizing principle for its data storage, analysis and user interface. It is inspired by the Design-Build-Test-Learn cycle of Total Quality and delivers data sets for interactive analysis and machine learning. Product developers reach holistic data-informed decisions with a 360 degree view of data across all workflows, from discovery through manufacturing processes.
“Riffyn’s technology has helped double the pace of R&D for our customers. Once you’ve had a chance to run one DoE successfully, you’ll be thinking, why hadn’t I done that sooner!” – Tim Gardner, CEO, Riffyn
Biopharma Life Cycle Value Chain
Process & Data Quality is the Foundation for Machine Learning
When you have fragmented, unusable data, users spend additional time on data cleaning and joining before analysis and machine learning. It slows the progress of science and impairs insight. A well-defined approach to digitization within the laboratory environment allows you to make full use of data to uncover new value and insights. Common pitfalls to avoid in your digital transformation journey include siloed data, lack of sample traceability, scattered and inaccessible data, data wrangling resulting from non-standard nomenclature, and lack of experimental design information.
Data Digitization Objectives for the Lab of the Future
Process Context
- Capture process parameters, materials, samples and time series data
- Standardize data format, naming, data access
Data Capture
- Rigorous tracking of data across the lifecycle from R&D to product
- Integrate and defragment data sets from files, instruments, LIMS and automation systems
Data Analytics
- Empower machine learning
- Compatibility with Minitab, JMP, other BI tools
Flexibility
- Workflow flexibility and scalability to grow
DoE Accelerates the Digital Pathway in Biopharma
Design of Experiments (DoE) is a revolutionary tool for characterizing multi complexity data that assists in building a continuous experimental model based upon past observations and predictive behavior. When combined with machine learning (ML) and artificial intelligence (AI) with a structured experimentation approach, DoE unlocks new ways to explore relationships within your data to support data driven decision making. It can transform your processes and permit a flexible, agile environment for scientific innovation.
But for DoE to work, having a connected flow of data is crucial. Riffyn Nexus fills that gap by providing the linking of process parameters from the DoE to the process context to the material and sample flow, and it places all the measured data into that context. This allows for a closed loop design-test-analyze cycle of learning without the usual challenges of finding and assembling data to support the DoE.
“It boils down to one simple lesson: in experimentation, we have to stop changing one variable at a time. It is a terribly inefficient and ineffective way to look at the complexity of nature’s mysteries”, says Tim Gardner, CEO of Riffyn.
Conclusion
Data is the number one asset of any R&D company. With the ever-increasing amount of data produced by life science R&D companies, knowledge harnessing and sharing within and across groups becomes significantly challenging. Data sources can become disparate. Better digital tools for collaborative data capture and analysis are more critical than ever to getting value from data assets. Such transparency in scientific processes across the entire biopharma value chain drive significant improvements in data quality and accessibility to accelerate product development.
Leveraging advanced tools such as DoE and machine learning hold the potential for transforming your operations into the digital lab of the future, if they are provided with such high-quality data. These tools provide the basis for shifting the traditional binary hypothesis-driven scientific approach towards a multivariate model-driven paradigm to enable the exploration of predictive modeling. Data can be aggregated and systematically analyzed to explore complex relationships to predict behavior and inform better, quicker decisions.
The Riffyn Nexus Process Data System provides the engine for driving such multivariate data analytics. It automatically structures, annotates, and integrates data from your data streams to reflect your experiments and workflows. Process and experiment data can be fed for real-time access to your data lakes and importation into business analytics and visualization software such as Spotfire, JMP, R and Python and DoE modeling applications.
Why it Matters for You
For predictive experimental models to work to your advantage, transparency in scientific processes across the entire biopharma value chain is crucial to improving data quality and accessibility.
- Optimizing the biopharma value chain workflow allows for transparency and accessibility across upstream and downstream processes and analytical chemistry to drive data-informed decision making.
- Shifting the paradigm towards a process-centric approach that places your R&D process flows, or ‘blueprints’, as the centralized hub of your laboratory ecosystem enables access to analysis-ready data in real-time for improved process lifecycle management.
- Incorporating Design of Experiment (DoE) for characterizing multivariate data will assist in building a continuous experimental model based upon past observations and predictive behavior.
- Implementing Riffyn Nexus in your R&D organization will provide analysis-ready data for use in any informatics application, enabling root cause analysis and DoE.
About Astrix
Astrix has been helping scientific organizations implement and integrate new informatics systems in the laboratory since 1995. Our experienced team of expert informatics consultants bring together technical, strategic, regulatory and content knowledge to provide the most effective solutions to problems faced by scientific organizations. Astrix partners with many of the industry leaders in the informatics space to offer state of the art solutions for all of your laboratory informatics needs. Our domain experts have helped hundreds of companies globally effectively navigate their digital transformation journey.
Case Study: LabWare Centralized Data Review for a Global Biopharmaceutical Company
Overview A global biopharmaceutical company specializing in discovery, development,... LEARN MOREWhite Paper: Managing Data Integrity in FDA-Regulated labs.
New White Paper LEARN MORELET´S GET STARTED
Contact us today and let’s begin working on a solution for your most complex strategy, technology and staffing challenges.
CONTACT US